Effective category-based clustering can help your business to optimize its assortment planning, inventory, and marketing management functions to reap the financial benefits of this exercise. However, before considering the clustering methods available to you, you first need to define your business goals and objectives.
The goals you choose must be in line with the method you select as well as with the availability of clustering tools such as access to data and clustering software. Some clustering methods are simple and require little resources or access to data. Others require a more complex approach.
This article will explore ten examples of clustering methods that can help you reach your business goals with reference made to the application, advantages, and disadvantages of each.
Cluster analysis tools
As a retailer, you have access to a variety of tools that you can use for cluster analysis. A few tools available include reports, datasheets, statistical clustering software, retail clustering software, and assortment implementation tools. As for how you choose the particular tool, that depends on the resources available to you as well as the goal of the clustering exercise you undertake.
Once you’ve created your clusters, the next step is to implement the results across your business, specifically within the functions of assortment planning, marketing, inventory management, and finance. The clustering method selected should facilitate simple integration of the cluster analysis results within your various business functions.
Your cluster metadata may provide information about the clusters such as the number of stores per cluster. It may also provide in-depth insights such as consumer purchasing patterns and pinpoint products that your customers typically purchase together. This information will help your retail buyers and other internal stakeholders to make data-driven strategic decisions.
Unpacking cluster analysis methods
Once you have determined which tools are available to conduct the clustering exercise, it’s time to consider the available clustering methods and decide which one suits your needs. As we mentioned above, the method you select must reflect the factors that are most in line with your overall goals.
For example, if you’d like to understand your consumers and want to better satisfy their needs and wants, a demographic-based clustering method may be most applicable. This applies to you regardless of if you’re a retailer or supplier.
On the other hand, you could implement clustering using a univariate (one variable) analysis such as climate. This approach is suitable when you have a specific organizational goal you want to achieve or one distinct influencing factor that causes differences between stores that stock a particular product category.
You can also create clusters using a multivariate (multiple variables) analysis. In this case, it requires a more advanced statistical analysis but can be more specifically tailored to the varying needs of your business.
Above is an example of multivariate clustering
Some clustering methods require little ongoing maintenance while others require a more dynamic approach to clustering that needs to be updated according to changes in consumer behavior and the industry in which your business operates. Meanwhile, some methods may be more appropriate for certain product categories than others.
It is also important to consider the integration and implementation of the cluster analysis.
Once you’ve received these results, you must make this information accessible to all of your business functions so that it can be used and implemented. So what are the different clustering methods available to you?
1. Single-Assortment Clusters
The first method is a single-assortment cluster. Here, each of your clusters receives the same product range. This approach is best suited to any retailers and suppliers who have a small assortment that is linked to the brand image.
If you are a retailer with a small number of store branches that serve a very similar target market, this would be a good approach. Apple would be a good example of this strategy. This method is easy to understand, requires very few resources, and is simple to execute across stores. However, it does not account for differing consumer demographics and product preferences across the market.
2. Channel-based clusters
The second method - channel-based clusters - sees each of your sales channels given its cluster and receiving a standardized product assortment.
This is a good preliminary approach to clustering as you can consider the unique characteristics of each of your sales channels. You have extra opportunities for profitability because fulfillment can occur across multiple channels with multiple assortments.
Generally, your online channel has the widest and deepest assortment while catalogs and brick-and-mortar stores have smaller offerings due to space restrictions. Brick-and-mortar stores may also have some in-store-only items where products are influenced by regulations or are impractical to sell online. This approach may be suitable for you if you run a single brick-and-mortar store and an online store.
That said, you may find that using this approach is the sub-optimization of the brick-and-mortar stores as you’ll cluster your product assortment across a channel rather than within a channel. This may occur because clustering does not even occur on a store level. Also, regional and local differences between customer preferences, demand, and LSM are not considered when using this approach.
3. Sales volume-based clusters
You can cluster your stores and categories based on historical and forecasted sales volume for a specified period. Known as a sales volume-based clustering method, it’s best suited to retailers and suppliers who have access to and want to use historical sales data for forecasting.
It’s also an easy approach to understand and implement because you can use product sales volume and performance data to expand, contract, and create your product range.
However, this approach can sometimes lead to an undifferentiated product offering. This is because buyers implementing this method typically use foot traffic, local competition, and customer density in the area to influence the product assortment. This method may also fail to consider the needs and wants of your target market.
4. Store capacity-based clusters
Stores and categories are grouped based on their available shelf and floor space. These figures may be measured in available floor space m², shelf space (length x height x depth), SKU count, or other detailed space planning metrics.
As far as clustering methods go, this particular approach helps your buyers determine the range cap for your assortment due to space constraints while being easy to understand and execute. However, it does not provide any information on which products you need to include in the range based on consumer needs and sales volume.
5. Sales volume and store capacity-based clusters
Stores/categories are clustered based on a combination of historical and forecasted data as well as capacity (available floor and shelf space). This method is best used if you have a similar customer base with slight differences in your product offerings as well as distinct differences in store sizes.
This method assists you and your buyers in determining the correct size of the product assortment while considering your capacity and performance data. However, this approach does not provide any insight into which products to include in your assortment. This method also may increase the number of clusters which increases the complexity of implementation of the results.
6. Climate or seasonality-based clusters
Described as a climate or seasonality-based clustering method, this approach is straightforward. In using it, you’d group your stores and categories based on seasonal weather patterns. It’s an approach that is most applicable if you carry seasonal products (e.g. produce or ski gear).
Before adopting this method, it is advised that you complete an in-depth data analysis with a focus on performance measures of seasonal products to justify this approach. You can then use the information you uncover to decide when to use this method, which products to include in the range, and when to add and remove them from your range.
7. Store format-based clusters
For this method, you’d group your store branches based on your store format (hypermarket, grocery store, convenience store, specialty store, and so on).
It’s also the type of cluster that you may base on either the store manager or customer feedback requesting specific types of products. For example, if you’re a convenience retailer, you may require products such as ready-made meals, charcoal, and long-life milk because consumers want to buy the essentials quickly and effortlessly. This method is most effectively used if local product requirements are consistent and clear for specific store branches.
Store format is generally identified and clustered based on performance data of particular products as well as location and demographic variables based on the needs of the end-user. This method is effective in optimizing localized demand. However, building an assortment plan for particular formats may require extra manual effort from you due to the vastly different target markets of each of your store formats.
8. Competition-based clusters
You can group store branches and categories on the presence and intensity of competition within the same market. While this method - competition-based clustering - is rarely used, may apply to you if you operate in an intense, saturated market.
This method may be beneficial to motivate you to create a specialty store format or offer deeper product assortments to encourage customer switching. However, it’s worth noting that this method does not assist you with assortment composition.
9. Demographic-based clusters
You also have the option to group your store branches and categories according to statistical demographic data. With this clustering method, you’d consider factors such as age, gender, monthly household income, and level of education to create your clusters. If you want to use this method, you can obtain demographic information through loyalty schemes (loyalty data) and external market data (from credit card companies and your suppliers).
This approach is beneficial because it allows you to understand your target market and build assortment plans that suit each cluster (e.g. specialized products for elderly people). However, you must understand the relationship between demographics and product preferences using data analysis.
10. Product attribute-based clusters
The final clustering method worth discussing is one where you’d group your stores and categories according to the historical performance data of specific products concerning product attributes.
In this case, you’d create clusters based on the composition of your assortment. A simple example is the category of fruit where you can have varieties of citrus fruit. If a particular store sells more oranges and another sells more lemons, these stores will fall within different clusters for the fruit category.
Once you created your cluster, you’d analyze the demographic data for each to determine the relationship between demographic and product attributes. If this relationship exists, it provides your buyers with information about your target market and allows them to make informed assortment decisions.
However, this approach can be difficult to understand, implement, and maintain across a business. Seasonality of products may also cause an assortment to change which will require the cluster analysis to be conducted regularly. Capacity is also not considered when this method is used, which may lead to a product range that is too large.
If you do decide to use this method, we recommend that the buyer create a master range for each cluster, which you’d need to cut down depending on the availability of shelf space in your store.
Conclusion
When assessing the above clustering methods and deciding which is right for you, DotActiv recommends a multivariate approach tailored to your business goals. Looking for assistance with your category-based clustering? Let DotActiv help. Find out more about our clustering services here or book a custom exploratory meeting here.
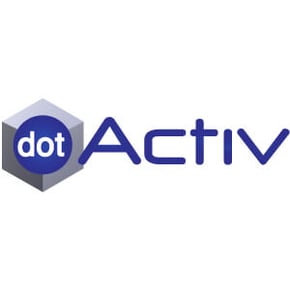