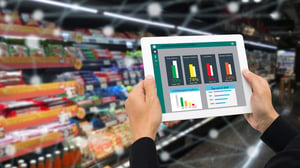
RETAIL DATA
Retail data refers to any facts or figures that retailers can collect about their business, which they can use to improve it.
It comes in many different shapes and forms, including point of sales data, loyalty card data, and market data.
TABLE OF CONTENTS
TABLE OF CONTENTS
WHAT IS RETAIL DATA?
Retail data refers to any facts or figures that retailers can collect about their business, which they can use to improve it.
It comes in many different shapes and forms, including point of sales data, loyalty card data, and market data.
There’s also your customer-centric data, supply chain and operations data and merchandising data. And harnessing all of this data is vital to the future of your business.
WHY IS RETAIL DATA IMPORTANT?
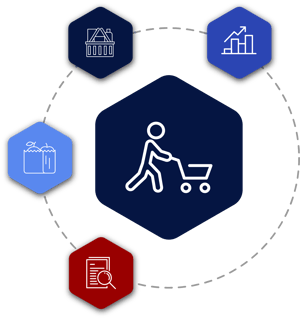
Considering the power that your customers have today - they can decide your store’s fate by choosing to either buy your products or not - you must remain a step ahead of them.
That is why retail data is so important. It gives you the information that you need to compete and compete well.
RETAIL DATA TERMINOLOGIES
It’s easy to get lost in all the terms and acronyms that surround data. It’s a complex topic. At its core, data is information in a raw or unorganised form. It includes numbers that refer to or represent conditions, ideas or objects.
Data is also limitless and present everywhere in the universe. In regards to computers, data are symbols or signals that are input, stored or processed by a computer for output as usable information.
COMMON DATA AND SPACE PLANNING TERMS
CAPS
Caps refers specifies the number of products that you can lay under or over the stack. When placing one item on top of another in a different orientation, it’s called a layover.
For example, if you have arranged cereal boxes on the shelf in a front end orientation - and sufficient headroom exists - you could place a box on top of the others in the front end orientation.
CUBIC SPACE
Cubic space calculates the volume or space of a product in cubic feet. You can use it to rationalise the amount of space given to a particular sub-category or segment of a product.
DAYS OF SUPPLY
Days of Supply (DOS) calculates the number of days a product will be on your shelf before replenishment is necessary. You can calculate DOS by dividing capacity by units sold per day.
FACINGS
Facings refer to the number of items with a single SKU on a shelf in linear measure. Giving your products the correct amount of facings plays a vital role in showing your customers that you can offer them what they want.
FORWARD SHARE
Forward share calculates the forward-sharing space a product occupies across the planogram. It is a relative measure for ensuring brands receive the forward-sharing space they deserve to entice a customer to purchase.
FRONTS
Fronts refer to the number of items that appear on the shelf above and behind a single facing.
MINIMUM DISPLAY DEPTH
You can use Minimum Display Depth (MDD) when you want to set a minimum limit to the number of products that can run deep into a shelf.
POG
Planogram (POG). A planogram is a diagram, drawing or visual representation that indicates how and where you should place your products on shelves.
UNIT OF MEASURE
UNIT OF MEASURE
Unit of Measure (UoM) is assigned to each product since it relates to the size measurement. The size of an item might be ‘500’. However, the UoM defines whether it’s grams, litres and so on.
WEEKLY MOVEMENT
Weekly movement is the number of units of a product that you sell each week. It indicates whether an item sells well or not so well. The weekly movement allows you to calculate your days of supply.
It is a measure that you must consider to understand your rate of sales and the overall movement of a product within your business.
CLEAN DATA
CLEAN DATA
Clean data is any data that you have reviewed. Before reviewing it, you will find it referred to as raw data. During the review, you should remove any replicated or duplicated data.
Each column should contain data. Missing or misspelt words within the columns will affect how the data is read or linked.
ETL
ETL is the acronym for Extract Transform Load. You would extract data using SQL before transforming it. This transformation includes data analysis, data cleaning, data standardisation and more.
Then, you would then integrate this data into a database. Database integration happens in various stages.
SQL
SQL is the acronym for Structured Query Language and is a computer language. You can use SQL to organise and simplify the process of adding, retrieving, editing, and deleting information stored in a database.
QUERY
A query is a complete select statement that specifies the columns and tables from which you retrieve data, conditions the data must satisfy, computations to perform on the retrieved column values, and a desired ordering of the result.
RELATIONAL DATABASE
A relational database organises data into tables and links them based on defined relationships. These relationships enable you to retrieve and combine data from one or more tables with a single query. SQL supports relational databases.
PRIMARY KEY
A primary key is a unique identifier you can use to draw links between each row in a table to draw the correct data.
You can also use it to identify a row of data in a specific table. You only have one primary key.
KEY
A key is a constructed column index that allows you rapid or sort access to a table row.
FOREIGN KEY
When there is a relation between two tables, you can connect these tables by inserting the primary key of one table into the corresponding row of the other table. The field used in such a way to connect the two tables is known as a foreign key.
RELATION
There are many cases where data in one table is related to data in another table. This connection between the two tables is known as a relation.
HOW IS RETAIL DATA STORED IN DOTACTIV?
DotActiv uses four tables, better known as dimensions. They are Market, Product, Period, and Fact.
For the software to function optimally, you need to identify the primary key in each dimension.
MARKET
The Market dimension depicts the information relating to the store.
It includes the following: Key Market Detail, Store Detail, Geographical Location and Cluster Detail.
KEY MARKET DETAIL
Key market detail is the primary key for the market dimension, and you cannot omit it from any data.
It is usually your Store Code or Store Name.
STORE DETAIL
Store detail includes specific store fields such as Store Name, Store Code, Retailer Name and Store Format.
You would base the format on your product variety, assortment, price, and location.
GEOGRAPHICAL LOCATION:
Geographical location refers specifically to the location of your store. It can include Country, Province, and Region.
It can also go further, including Building Number, City, GPS Coordinates, Postal Address, Region Code, Street, and more.
You must select the right store and format as the range or assortment may differ accordingly.
CLUSTER DETAIL
Cluster detail refers to the grouping of similar stores and includes Cluster Name, Cluster Size, and Cluster Type.
You can also include Cluster Description and Cluster Number.
PRODUCT
The Product dimension includes all information relating to the product.
It includes the following: Key Product Detail, Item Detail, Hierarchy and Dimensions.
KEY PRODUCT DETAIL
Key product detail is the primary key for the product dimension, and you cannot omit it from any data.
It will be your Barcode or the Article Code as it is unique and unreplicable.
ITEM DETAIL
Item detail includes all the fields related to your product such as Barcode, Brand, Product Description, Product Code, Size and UOM, and Supplier.
HIERARCHY
Hierarchy refers specifically to the classification of your products. It includes Supergroup A, Supergroup B, Category, Sub-Category, Segment, and Sub-Segment.
Your products must be classified since it ensures that your planograms keep related items together and in the correct flow.
DIMENSIONS
This refers to the actual dimensions of your product, namely your height, width, and depth.
It is a critical dataset for creating visually appealing and correct planograms.
PERIOD
The Period dimension allows you to report on the time frame chosen and includes Period From and Period To.
Both of these are primary keys.
FACT
The Fact dimension includes data that you cannot alter and includes your sales and units. While the Market, Product, and Period dimensions are all flexible, for Fact, you need sales and units data.
Facts include Retail, Indicators, Stock, and Space Planning.
RETAIL
Retail, here, includes fields related to the sale of your product, including Sales, Units, % Sales, % Units, Cumulative % Sales, and Cumulative % Units.
Cumulative will identify which top 20% of your products draws in 80% of your Sales or Units.
INDICATORS
Your indicators would be used for your ranging exercises and include fields such as Core Range, Ranging Indicators, and Buyers Indicators.
STOCK
All of your fields for stock control are here, including Stock Value At Cost, Minimum Stock, Lead Time, and Case in DC. DC refers to your Distribution Centre.
It is included in an environment that is integrated with the distribution centre to show available stock levels.
SPACE PLANNING
Space planning indicates all the fields you need to optimise your space. Examples include DOS, Forward Share, Actual Facings and Weekly Movement. DotActiv usually formulates these statistics for you.
DATA TRANSFER METHODS
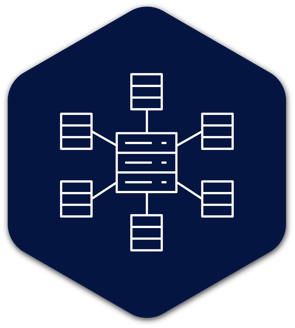
The transfer of data is, as PC Mac describes it, the “copying [of] data from one computer to another. When a network is used, data are technically ‘transmitted’ over the network, rather than transferred; however, the terms transfer and transmit are used synonymously”.
When the time comes to transfer data, you have several options available to you. Below are two examples:
Transact-SQL: Transact-SQL is Microsoft and Sybase’s extension of SQL, and you can use it to send data between relational databases. This method requires the ability to write SQL scripts or queries using Transact-SQL.
DotActiv Data Import Utility: DotActiv has a dedicated application that imports data from SQL staging tables into the DotActiv data platform. Once set up, you can schedule the import utility to run its imports at whatever time you choose. The data import utility can run automatically.
TRANSFERRING DATA TO DOTACTIV'S DATA PLATFORM
At DotActiv, we use Microsoft SQL, which supports any versions of SQL Server from 2014 and onwards. When it comes to transferring data, you can approach our category management platform in two ways:
1. By manually preparing and uploading datasets using CSV and Excel files on an ad-hoc basis. It is available for all our solutions, from DotActiv Lite to Dotactiv Enterprise.
2. Through automation by integrating DotActiv into your business. You can achieve this integration by using staging databases and Transact-SQL statements or queries via the DotActiv data import utility. It is only available in DotActiv Professional and DotActiv Enterprise.
DATA SOURCES
WHAT ARE DATA SOURCES?
A data source is primarily a location from which you can use data. Better put, it is a collection of records that store data that you can use in the future or when needed.
Concerning a retail business, that could be any data related to the products you sell in your store, any data on the number of sales you make, or on your overall stock.
From a simple file to a complex database on a database management system to a live data feed, data sources take various forms. As for where data is stored, there are only two instances. You would either store data on the same computer as the program you are trying to access. Or, you would store it on a different computer on your network.
DATA SOURCE EXAMPLES
CSV FILES
Colloquially known as CSV’s, you would use a comma-separated values file to move tabular data (numbers and text) between programs that operate on incompatible formats.
For example, you may need to transfer information from an application, which stores data in a particular format to a spreadsheet that uses an entirely different format.
Similarly to Excel, getting data from a CSV file into DotActiv is straightforward and does not require specialist skills.
MICROSOFT EXCEL
Microsoft Excel is often referred to as the global standard for spreadsheets since it is compatible with just about any Operating System.
A few main features include Calculations or Basic Math, Charts, Pivots Tables, and Conditional Formatting.
Obtaining data from Excel into DotActiv is straightforward and does not require any specialist skills.
DATA WAREHOUSE
A data warehouse is similar to any retail warehouse you would usually visit. The only difference is that while your usual warehouse may contain a multitude of merchandise, this one stores current and historical data.
The most common tools used as a data warehouse includes Microsoft SQL and Oracle - a data warehouse is a function, not the tool itself.
To extract data from any source system into the data warehouse, you can use a process known as ETL - extraction, transformation, and loading.
STAGING DATABASE
At its core, a staging database is an intermediate storage area for your data. From this location, you can extract your data.
The primary purpose of your staging database is to serve as a working area where you can organise your data into a format. Then, you can match it to a target application. You can also refer to these as staging tables, landing zones or staging areas.
POINT OF SALE DATA
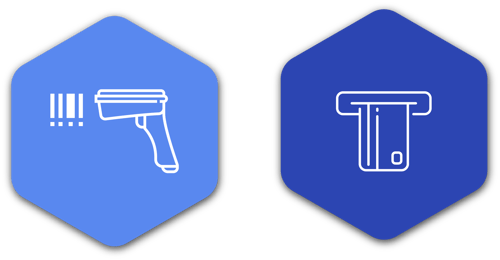
WHAT IS POS DATA?
Point of sale (POS) refers to the area where an in-store transaction occurs. Better put, it is where your staff scan and bag goods and where your customers pay.
As for your point of sale data, you can collect it directly from your retail POS system, which includes a combination of software and hardware.
Regardless of the size of your stores, your POS system should include a computer, monitor, cash drawer, receipt printer, customer display and barcode scanner. It is also worth investing in a Credit or Debit Card reader.
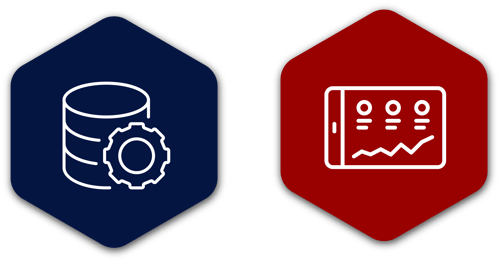
THE POWER OF POS DATA
There is a power that comes with collecting POS data. And it’s not just DotActiv who says that. It’s also a point that Jake Freivald, vice president of corporate marketing at the software company, Information Builders, makes.
He’s quoted by Mobiquity, saying, “Point-of-sales data is the lifeblood of a retail company. It’s probably more important than any other single data source, because it helps us understand the past, monitor the present and predict the future.”
With this data captured, you will have a better picture of your overall sales, the daily, weekly, monthly and event yearly movement of your products, and whether your sales tactics are working. And that is just the start.
In a POS data white paper, data-as-a-service platform, StrikeIron, points out that it can also help you build and drive customer relationships.
LOYALTY CARD DATA
WHAT IS LOYALTY CARD DATA?
Loyalty cards, and the customer data you can collect from them, can influence how you run your business. That is because it will allow you to know your shopper. And with this type of retail analytics data at your disposal, you can only expect to profit.
Similar to POS data, there is also power in loyalty card data. It can tell you the reasons why your shoppers are coming back to your store. It can also tell you how much your shoppers are spending on average per trip.
More than that, though, is what you can do with this data. And that is where its true power lies.
To make full use of the data you collect from your loyalty cards, you need to plug it back into your business. One way of doing that is to use it to build your ranging strategies.
IT TELLS YOU WHICH STRATEGIES TO CONSIDER FOR GROWTH
In looking at the basket behaviour of your customers, you can see what they are buying and align your strategies with that information.
IT TELLS YOU WHICH SEGMENTS TO PRIORITISE
Based on the information gathered from your shopper profiles, you can understand where you need to prioritise.
IT TELLS YOU WHICH MUST-STOCK SKUS YOU NEED
This is data based on your SKU performance. In short, it allows you to decide which SKUs have low levels of transferable demand and which are worth leaving off your list.
MARKET DATA
WHAT IS MARKET DATA?
Retailers and suppliers often enter into data exchange agreements with third-party market data providers. In this process, they share certain information about their own business with the third party data provider in return for information about the market in which they operate.
DotActiv’s software uses both internal data and external market data when calculating assortments and ranges.
Here is an example as to why this is of critical importance:
If a product performs poorly in your stores while simultaneously performing well in the market, the solution is not necessarily to delist the product. Instead, you can look at why the product is not selling as expected in your stores.
RETAIL ANALYTICS
WHAT IS RETAIL ANALYTICS?
As a concept, retail analytics can become very technical very quickly. That means it is easy to throw out a technical definition, which would involve all sorts of factors. But it is not necessary.
Retail analytics refers to a set of techniques you can use to observe and analyse all things concerning your store. As a result, you can make smarter decisions to manage your business better.
On a technical level, it provides analytical data on several factors around your business, including:
INVENTORY LEVELS
Having the necessary products in the right quantity at the right time will help your store to maintain an optimal inventory level
You can also understand what items you should stock and which items you should not.
SUPPLY CHAIN MOVEMENT
You’ll have access to information about the movement of every product that you have within your store.
CUSTOMER DEMANDS
By looking at your collected data, you can learn more about customer behaviour and understand where product demand lies.
That will inform your assortment planning, which will ensure you have the most valued SKUs or items listed and placed in easy to find locations within your store.
SALES FIGURES
Without efficient analytical data, you won’t be able to prove if what you’re doing is helping your bottom line or not.
WHY DO RETAIL ANALYTICS MATTER SO MUCH?
In the past, selling to the public was less complicated. Back then, retailers controlled the buying process, telling consumers what they needed, when they needed it, and where they could get it.
Today, however, customers are empowered and have a list of demands that you need to meet. They want you to make it easier for them to interact with you. They want you to be able to be available when and if they need you. And at any time. They want you to cater for their every whim.
With good data on your side, the above should not be as intimidating as it sounds.
RETAIL ANALYTICS BENEFITS
You can understand the sales contribution of all of your categories, sub-categories, segments and SKUs. You can also know how they perform in terms of value.
You can understand the units contribution of all of your categories, sub-categories, segments and SKUs. You can also know how they perform in terms of value. Lastly, it helps you strategically place yourself in the marketplace.
You can identify who your target market is, which allows you to align your assortments with that market.
THE DIFFERENT TYPES OF RETAIL ANALYTICS
With the correct type of data, your business has the power to gain a competitive edge. To ensure that happens, you have to have more than just the necessary data. You also need to understand the different types so you can leverage the data.
There are four different types of data, namely Descriptive, Diagnostic, Predictive, and Prescriptive. And each of them answers a question, which helps your business to move forward.
DESCRIPTIVE
Descriptive analytics is the simplest form of analytics. It analyses incoming data for insights on how to move forward. In short, it looks at the past so that you can be better prepared for the future.
It answers the question, ‘What happened?’ and looks at the reason/s behind your success or failure.
DIAGNOSTIC
Diagnostic analytics digs deeper into the data to better understand what caused the behaviour. With it, you attempt to determine where there are problems in your pipeline and why.
It answers the question, ‘What did it happen?’ and helps you to isolate the root cause of the problem.
PREDICTIVE
It's critical to understand what has happened and why if you want to move forward. But it is just as important to look at what will happen. And this is what you get with predictive analytics.
It answers the question, ‘What will happen?’ and focuses on forecasting.
It's worth noting that this type of analytics will not tell you what will happen - no analytics can do that. But it can provide you with an answer to questions that you cannot answer with business intelligence
PRESCRIPTIVE
This type of analytics goes far beyond forecasting by simulating the future under various sets of assumptions.
It answers the question, ‘How can we make it happen?’ And in so doing, it anticipates not only what will happen and when it will happen but also why it will happen.
It is complex to manage, which means that not many companies use it in their day-to-day operations.
GETTING STARTED WITH RETAIL ANALYTICS
If you are looking to get started with retail analytics, the best place to start would be by setting a goal to cover the basics while setting up your infrastructure. Thus, when moving to more advanced retail analytics, you would not have limited yourself.
To achieve any goal that you choose, you must follow these six steps:
1. DEFINE THE QUESTIONS THAT YOU SEEK TO ANSWER
Before you begin identifying your data sources or anything else, you must first think carefully about the questions you want to answer and then write them down.
For example, within your top-performing categories, which sub-categories and segments are showing a consistent growth trend?
In defining your question, you’re stopping yourself from getting lost in too much information.
2. IDENTIFYING YOUR DATA SOURCES
After defining your questions, it’s time to identify where you are going to get your data.
Are the required fields in your data warehouse, ERP or POS applications?
3. IDENTIFY WHAT TECHNOLOGY IS REQUIRED
You need a data platform, hardware to host the data, a data transfer method, and a tool from which you will create custom retail analytics.
Excluding the hardware component, both DotActiv Professional and Enterprise cover these requirements comprehensively.
4. TRANSFER DATA
It’s now time to transfer your data from your sources to your platform.
If you’re interested in DotActiv as a solution, it’s worth noting that our team of experts will set up your data transfers to occur automatically on your behalf.
5. CLEAN AND CLASSIFY YOUR DATA
Once you have transferred your data to your data platform, it’s critical to ensure that your data is clean.
If we go back to the example we used above, ensure that you have classified each product line item into the most appropriate category, sub-category, segment, and sub-segment.
6. CREATE YOUR DASHBOARDS
This is your final step, and it’s where all the magic comes to life. DotActiv Professional and Enterprise both come with a set of best practice retail analytics reports that seek to answer the most popular questions asked.
If you have not answered any of your questions yet, you can create customised reports and a category management dashboard.
GET STRATEGIC CATEGORY MANAGEMENT ADVICE UNIQUE TO YOUR BUSINESS
With DotActiv’s retail advisory services, if you’re a retailer, you can get strategic category management advice that helps you to improve your business.
If you’re a supplier, this advice can help you improve your product exposure through increase shelf space allocations.
We can also help you to create or crystallise your short, medium and long-term category management vision.
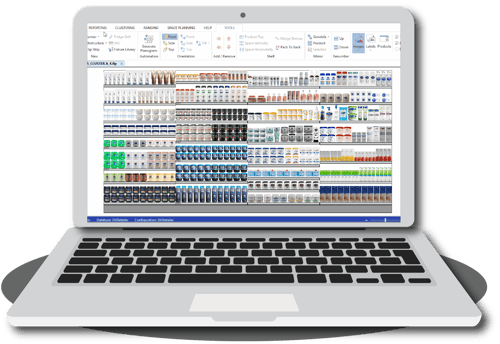